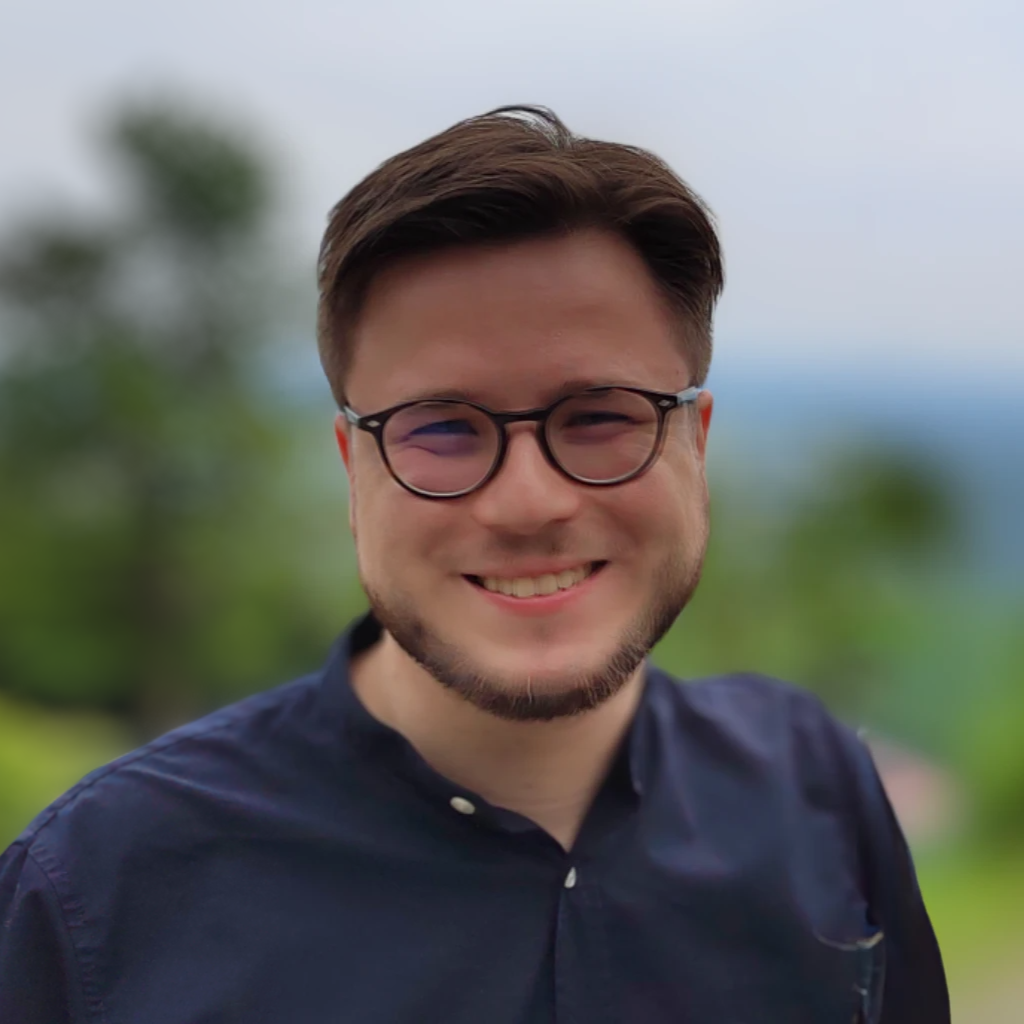
whoami
🤓
I’m Emanuele, a PhD student in neuro-inspired and robust Deep Learning — part of the Applied Data Science and Artificial Intelligence doctoral programme — among the Artificial Intelligence Laboratory (AILab) at the University of Trieste, Italy.
Previously, I earned a MSc in Data Science and Scientific Computing (2022) and a BSc in Physics (2020), both from the University of Trieste.
Currently, I’m applying Deep Learning methods inspired by cognitive neuroscience and neurobiology (e.g. in the form of generative modelling and implicit manifold learning) to the improvement of efficiency, effectiveness, and adversarial robustness of neural image classifiers.
I am supervised by Luca Bortolussi (AILab, UniTS) and collaborate with Alessio Ansuini (LADE, AREA Science Park), Fabio Anselmi (MLCSL, UniTS), and Alex Rodriguez (UniTS).
Research interests (TL;DR: 🧠 ⇌ 💻)
My research interests broadly comprise:
- Adversarially-robust Deep Learning
Current direction(s): purification-based and generative adversarial defences, genesis of adversarial robustness and lack thereof, Bayesianity as a proxy to adversarial robustness in artificial neural networks. - Phenomenology and experimental science of deep artificial neural networks
Current direction(s): implicit biases of artificial neural network architectures, efficient learning with random and sampled features, effects of data geometry on learning dynamics and learned representations, phase transitions. - Conditional generative modeling: variational and diffusive.
- Kernel methods and manifold learning techniques in Deep Learning.
- Atypical approaches to Deep Learning and Artificial Intelligence.
- Translational Artificial Intelligence: transferring insights from neuro ‑science, ‑biology, ‑physiology and ‑psychology to artificial intelligence.
Current direction(s): synthetic foveation in Deep Learning for computer vision.
Moonshot goals: closer-to-biological artificial intelligence, AGI. 🚀
Alia 🗣️
When not about any of the above, I usually discuss about optimality in policy-making and electoral systems, energy policy (since way before it was everyday matter!), and open science/software/knowledge which I try to embrace, support and promote.
I am also serving as part of the Directive Committee of the Artificial Intelligence Student Society, OdV, an outreach-centric volunteer organization operating chiefly in the Trieste Area (whose core interest should be clear from the name).